PERCEPTRON
1. In 1957, Rosenblatt
and several other researchers developed perceptron, which used the similar
network as proposed by McCulloch, and the learning rule for training network to
solve pattern recognition problem.
(*) But,this model was later criticized by Minsky
who proved that it cannot solve the XOR problem.
2. The network structure:
3. The training process:
Choose the network layer,nodes,& connections
Randomly assign weights: W
& bias: 
Input training sets X
(preparing T
for verification )
Training computation:
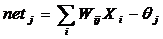
(7) Recall : after the network has trained
as mentioned above, any input vector X can be send into the perceptron
network. The trained weights,
, and the bias,
, is used to derive
and, therefore, the output
can be obtained for
pattern recognition.
Ex: Solving the OR problem
Let the
training patterns are used as follow.
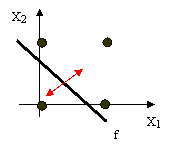
X1 X2
|
T
|
0 0
|
0
|
0 1
|
1
|
1 0
|
1
|
1 1
|
1
|
Let W11=1,
W21=0.5, Θ=0.5
The initial net function is:
net = W11X1+ W21X2-Θ η=0.1
net = 1X11
+ 0.5X21 - 0.5
Feed the input pattern into network one by one
(0,0), net= -0.5, Y=0, δ= 0
O.K.
(0,1), net= 0, Y= 0, δ=1- =1 (need to update weight)
(1,0) net=
0.5, Y=1, δ= 0 O.K.
(1,1) net=
1, Y=1, δ= 0 O.K.
update
weights for pattern (0,1) which is not satisfying the expected output:
ΔW11=(0.1)(1)( 0)= 0, ΔW12=(0,1)(1)( 1)= 0.1, ΔΘ=-(0.1)(1)=-0.1
W11=
W11+ΔW11=1, W21=0.5+0.1=0.6, Θ=0.5-0.1=0.4
Applying new weights to the net function:
net=1X1+0.6X2-0.4
Verify the pattern (0,1) to see if it satisfies the expected
output.
(0,1), net= 0.2, Y= 1, δ=
Feed the next input pattern, again, one by one
(1,0), net= 0.6, Y=1, δ=
(1,1) , net= 1.2, Y=1, δ=
Since the first pattern(0,0) has not been testified with the
new weights, feed again.
(0,0), net=-0.4, Y=, δ=
Now, all the patterns are
satisfied the expected output. Hence,
the network is successfully trained for understanding the OR problem(pattern).
We can generate the pattern recognition function for OR
pattern is:
net= X1
+ 0.6X2 -
0.4 (This is not the only solution, other solutions
are possible.)
The trained network is formed as
follow:
Recall
process:
Once the network is trained, we
can apply any two element vectors as a pattern and feed the pattern into the
network for recognition. For example, we
can feed (1,0) into to the network
(1,0), net= 0.6,
Y=1 Therefore, this pattern is
recognized as 1.
Ex: Solving the AND problem (i.e., recognize the AND
pattern)
Let the training
patterns are used as follow.
X1 X2
|
T
|
0 0
|
0
|
0 1
|
0
|
1 0
|
0
|
1 1
|
1
|
Let W11=0.5, W21=0.5, Θ=1,
Let η=0.1
The initial net function is:
net =0.5X11+0.5X21 1
Feed the input pattern into network one by one
(0,0), net=-1 Y=, δ=
(0,1), net=-0.5 Y= , δ=
(1,0) net=- 0.5, Y= , δ=
(1,1) net= , Y= , δ= 1
update
weights for pattern (1,1) which does not satisfying the expected output:
ΔW11=(0,1)(1)( 1)= 0.1,
ΔW21=(0,1)(1)( 1)= 0.1,
ΔΘ=-(0.1)(1)=-0.1
W11=0.6, W21=0.5+0.1=0.6, Θ=1-0.1=0.9
Applying new weights to the net function:
net=0.6X1 + 0.6X2 - 0.9
Verify the pattern (1,1) to see if it satisfies the expected
output.
(1,1) net= 0.3, Y= 1 , δ=
Since the previous patterns are not testified with the new
weights, feed them again.
(0,0), net=-0.9 Y=, δ=
(0,1), net=-0.3 Y= , δ=
(1,0) net=- 0.3, Y= , δ=
We can generate the pattern recognition function for OR
pattern is:
net= 0.6X1
+ 0.6X2 - 0.9 (This is not the only solution, other solutions
are possible.)
The
trained network is formed as follow:
Ex: Solving the XOR problem
Let the training
patterns are used as follow.
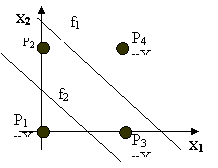
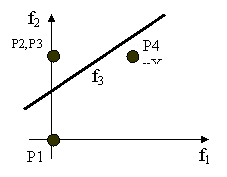
X1 X2
|
T
|
0 0
|
0
|
0 1
|
1
|
1 0
|
1
|
1 1
|
0
|
Let W11=1.0, W21= -1.0, Θ=0,
If
we choose one layer network, it will
be proved that the network cannot be
converged. This is because the XOR
problem is a non-linear problem, i.e.,
one single linear function is not enough
to recognize the pattern. Therefore, the
solution is to add one hidden layer for extra
functions. The
following pattern is formed.
X1 X2
|
T1 T2
|
T3
|
0 0
|
0 0
|
0
|
0 1
|
0 1
|
1
|
1 0
|
0 1
|
1
|
1 1
|
1 1
|
0
|
Let W11=0.3, W21=0.3, W12= 1, W22= 1
The initial net function for node f1 and node f2 are:
f1 = 0.3X11+ 0.3X21 - 0.5
f2 = 1X12+
1X22 - 0.2
Now we need to feed the input one by one for training the
network.for f1 and f2 seprearately. This
is to satisfiying the expected output for f1 using T1 and for f2 using T2.
Finally, we use f1 and f2 as input pattern to train the node
f3, the result is
f3=1X13 - 0.5X23 + 0.1